As part of my role the Python advocacy team for Azure, I am now one of the maintainers on several ChatGPT samples, like my simple chat app and this popular chat + search app. In this series of blog posts, I'll share my learnings for writing chat-like applications. My experience is from apps with Python backends, but many of these practices apply cross-language.
Today I want to talk about the importance of streaming in the UI of a chat app, and how we can accomplish that. Streaming doesn't feel like a must-have at first, but users have gotten so accustomed to streaming in ChatGPT-using interfaces like ChatGPT, Bing Chat, and GitHub CoPilot, that they expect it in similar experiences. In addition, streaming can reduce the "time to first answer", as long as your UI is calling the streaming OpenAI API as well. Given it can take several seconds for ChatGPT to respond, we welcome any approaches to answer user's questions faster.

Streaming from the APIs
The openai package makes it easy to optionally stream responses from the API, by way of a stream
argument:
chat_coroutine = await openai_client.chat.completions.create(
deployment_id="chatgpt",
model="gpt-3.5-turbo",
messages=[
{"role": "system", "content": "You are a helpful assistant."},
{"role": "user", "content": request_message},
],
stream=True,
)
When stream
is true, the response type is an asynchronous generator, so we can use async for
to process each of the ChatCompletion chunk objects:
async for event in await chat_coroutine:
message_chunk = event.choices[0].delta.content
Sending stream from backend to frontend
When we're making a web app, we need a way to send those objects as a stream from the backend to the browser. We can't use a standard HTTP response, since that sends everything at once and closes the connection. The most common approaches for streaming from backends are:
- WebSockets: Bidirectional communication channel, client or server can push.
- Server-sent events: An HTTP channel for server to push to client.
- Readable streams: An HTTP response with a
Transfer-encoding
header of "chunked", signifying the browser must wait for all chunks.
All of these could potentially be used for a chat app, and I myself have experimented with both server-sent events and readable streams. Behind the scenes, the ChatGPT API actually uses server-sent events, so you'll find code in the openai package for parsing that protocol. However, I now prefer using readable streams for my frontend to backend communication. It's the simplest code setup on both the frontend and backend, and it supports the POST requests that our apps are already sending.
The key is to send the chunks from the backend using the NDJSON (jsonlines) format, and parse that format in the frontend. See my blog post on fetching JSON over streaming HTTP for Python and JavaScript example code.
Achieving a word-by-word effect
With all of that implemented, we have a frontend that reveals the answer gradually:

Here's what's interesting: despite our frontend receiving chunks of just a few tokens at a time, it appears to reveal almost entire sentences at a time. Why does the frontend UI seem to stream much larger chunks than what it receives? That's likely caused by the browser batching up repaints, deciding that it can wait to display the latest update to the innerHTML of the answer element. Normally that's a great performance enhancement on the browser's side, but it's not ideal in this case.
My colleague Steve Steiner experimented with various ways to force the browser to repaint more frequently, and settled on a technique that uses window.setTimeout()
with a delay of 33 milliseconds for each chunk. That does mean that the browser takes overall more time to display a streamed response, but it doesn't end up faster than reading speed. See his PR for implementation details.
Now the frontend displays the answer at the same level of granularity that it receives from the chat completions API:
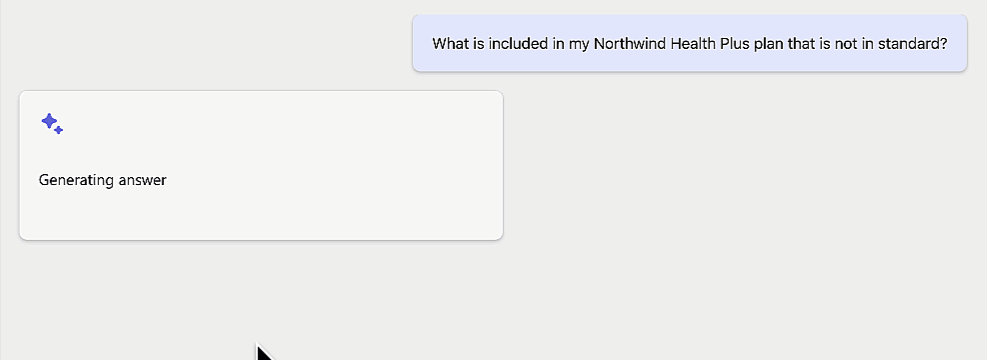
Streaming more of the process
Many of our sample apps are RAG apps that "chat on your data", by chaining together calls across vector databases (like Azure Cognitive Search), embedding APIs, and the chat completions API. That chain of calls will take longer to process than a single ChatCompletion call, of course, so users may end up waiting longer for their answers.
One suggestion from Steve Steiner is to stream more of the process. Instead of waiting until we had the final answer, we could stream the process of finding the answer, like:
- Processing your question: "Can you suggest a pizza recipe that incorporates both mushroom and pineapples?"
- Generated search query "pineapple mushroom pizza recipes"
- Found three related results from our cookbooks: 1) Mushroom calzone 2) Pineapple ham pizza 3) Mushroom loaf
- Generating answer to your question...
- Sure! Here's a recipe for a mushroom pineapple pizza...
We haven't integrated that idea into any of our samples yet, but it's interesting to consider for anyone building chat apps, as a way to keep the user engaged while the backend does additional work.
Making it optional
I just spent all that time talking about streaming, but I want to leave you with one final recommendation: make streaming optional, especially if you are developing a project for others to deploy. There are some web hosts that may not support streaming as readily as others, so developers appreciate the option to turn streaming off. There are also some use cases where streaming may not make sense, and it should be easy for developers (or even users) to turn it off.
No comments:
Post a Comment