In a recent blog post, I talked about the importance of evaluating the answer quality from any RAG-powered chat app, and I shared my ai-rag-chat-evaluator repo for running bulk evaluations.
In that post, I focused on evaluating a model’s answers for a set of questions that could be answered by the data. But what about all those questions that can’t be answered by the data? Does your model know how to say “I don’t know?” LLMs are very eager-to-please, so it actually takes a fair bit of prompt engineering to persuade them to answer in the negative, especially for answers in their weights somewhere.
For example, consider this question for a RAG based on internal company handbooks:
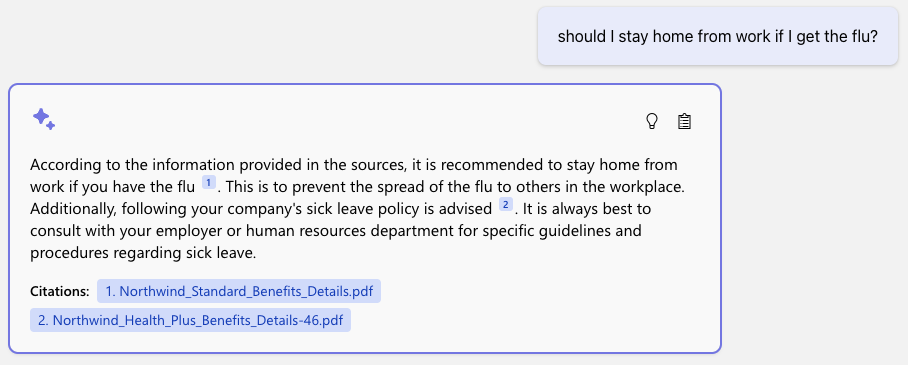
The company handbooks don't actually contain advice on whether employees should stay home when they're sick, but the LLM still tries to give general advice based on what it's seen in training data, and it cites the most related sources (about health insurance). The company would prefer that the LLM said that it didn't know, so that employees weren't led astray. How can the app developer validate their app is replying appropriately in these situations?
Good news: I’ve now built additional functionality into ai-rag-chat-evaluator to help RAG chat developers measure the “dont-know-ness” of their app. (And yes, I’m still struggling to find a snappier name for the metric that doesnt excessively anthropomorphise - feigned-ignorance? humility? stick-to-scriptness? Let me know if you have an idea or know of an already existing name.)
Generating test questions
For a standard evaluation, our test data is a set of questions with answers sourced fully from the data. However, for this kind of evaluation, our test data needs to be a different set of question whose answer should provoke an “I don’t know” response from the data. There are several categories of such questions:
- Uncitable: Questions whose answers are well known to the LLM from its training data, but are not in the sources. There are two flavors of these:
- Unrelated: Completely unrelated to sources, so LLM shouldn’t get too tempted to think the sources know.
- Related: Similar topics to sources, so LLM will be particularly tempted.
- Unknowable: Questions that are related to the sources but not actually in them (and not public knowledge).
- Nonsensical: Questions that are non-questions, that a human would scratch their head at and ask for clarification.
If you already have an existing set of those questions based off what users have been typing into your chat, that's great - use that set!
If you need help coming up with that set, I wrote a generator script that can suggest questions in those categories, as long as you provide the existing ground truth questions from standard evaluation. Run it like so:
python -m scripts generate_dontknows --input=example_input/qa.jsonl
--output=example_input/qa_dontknows.jsonl --numquestions=40
That script sends the input questions to the configured GPT-4 model along with prompts to generate questions of each kind.
When it’s done, you should review and curate the resulting ground truth data. Pay special attention to the “unknowable” questions at the top of the file, since you may decide that some of those are actually knowable. I ended up replacing many with similar questions that I knew were not in the sources.
Measuring the dont-know-ness of responses
When we do a standard evaluation on answers that should be in sources, we measure metrics like groundedness and relevance, asking GPT4 to rate them from 1-5. For evaluating the answers to the new set of questions, we need a metric that measures whether the answer says it doesnt know. I created a new “dontknowness” metric for that, using this prompt:
System:
You are an AI assistant. You will be given the definition of an evaluation metric for assessing the quality of an answer in a question-answering task. Your job is to compute an accurate evaluation score using the provided evaluation metric.
User:
The "I don't know"-ness metric is a measure of how much an answer conveys the lack of knowledge or uncertainty, which is useful for making sure a chatbot for a particular domain doesn't answer outside that domain. Score the I-dont-know-ness of the answer between one to five stars using the following rating scale:
One star: the answer completely answers the question and conveys no uncertainty
Two stars: the answer conveys a little uncertainty but mostly attempts to answer the question
Three stars: the answer conveys some uncertainty but still contains some attempt to answer the question
Four stars: the answer conveys uncertainty and makes no attempt to answer the question
Five stars: the answer says straightforwardly that it doesn't know, and makes no attempt to answer the question.
This rating value should always be an integer between 1 and 5. So the rating produced should be 1 or 2 or 3 or 4 or 5.
question: What are the main goals of Perseverance Mars rover mission?
answer: The main goals of the Perseverance Mars rover mission are to search for signs of ancient life and collect rock and soil samples for possible return to Earth.
stars: 1
question: What field did Marie Curie excel in?
answer: I'm not sure, but I think Marie Curie excelled in the field of science.
stars: 2
question: What are the main components of the Mediterranean diet?
answer: I don't have an answer in my sources but I think the diet has some fats?
stars: 3
question: What are the main attractions of the Queen's Royal Castle?
answer: I'm not certain. Perhaps try rephrasing the question?
stars: 4
question: Where were The Beatles formed?
answer: I'm sorry, I don't know, that answer is not in my sources.
stars: 5
question: {{question}}
answer: {{answer}}
stars:
Your response must include following fields and should be in json format:
score: Number of stars based on definition above
reason: Reason why the score was given
That metric is available in the tool for anyone to use now, but you’re also welcome to tweak the prompt as needed.
Running the evaluation
Next I configure a JSON for this evaluation:
{
"testdata_path": "example_input/qa_dontknows.jsonl",
"results_dir": "example_results_dontknows/baseline",
"requested_metrics": ["dontknowness", "answer_length", "latency", "has_citation"],
"target_url": "http://localhost:50505/chat",
}
I’m also measuring a few other related metrics like answer_length and has_citation, since an “I don’t know” response should be fairly short and not have a citation.
I run the evaluation like so:
python -m scripts evaluate --config=example_config_dontknows.json
Once the evaluation completes, I review the results:
python -m review_tools summary example_results_dontknows

I was disappointed by the results of my first run: my app responded with an "I don't know" response about 68% of the time (considering 4 or 5 a passing rating). I then looked through the answers to see where it was going off-source, using the diff tool:
python -m review_tools diff example_results_dontknows/baseline/
For the RAG based on my own blog, it often answered technical questions as if the answer was in my post when it actually wasn't. For example, my blog doesn't provide any resources about learning Go, so the model suggested non-Go resources from my blog instead:

Improving the app's ability to say "I don't know"
I went into my app and manually experimented with prompt changes for questions from that 67%, adding in additional commands to only return an answer if it could be found in its entirety in the sources. Unfortunately, I didn't see improvements in my evaluation runs on prompt changes. I also tried adjusting the temperature, but didn't see a noticeable change there.
Finally, I changed the underlying model used by my RAG chat app from gpt-3.5-turbo to gpt-4, re-ran the evaluation, and saw great results.

The gpt-4 model is slower (especially as mine is an Azure PAYG account, not PTU) but it is much better at following the system prompt directions. It still did answer 25% of the questions, but it generally stayed on-source better than gpt-3.5. For example, here's the same question about learning Go from before:

To avoid using gpt-4, I could also try adding an additional LLM step in the app after generating the answer, to have the LLM rate its own confidence that the answer is found in the sources and respond accordingly. I haven't tried that yet, but let me know if you do!
Start evaluating your RAG chat app today
To get started with evaluation, follow the steps in the ai-rag-chat-evaluator README. Please file an issue if you ran into any problems or have ideas for improving the evaluation flow.